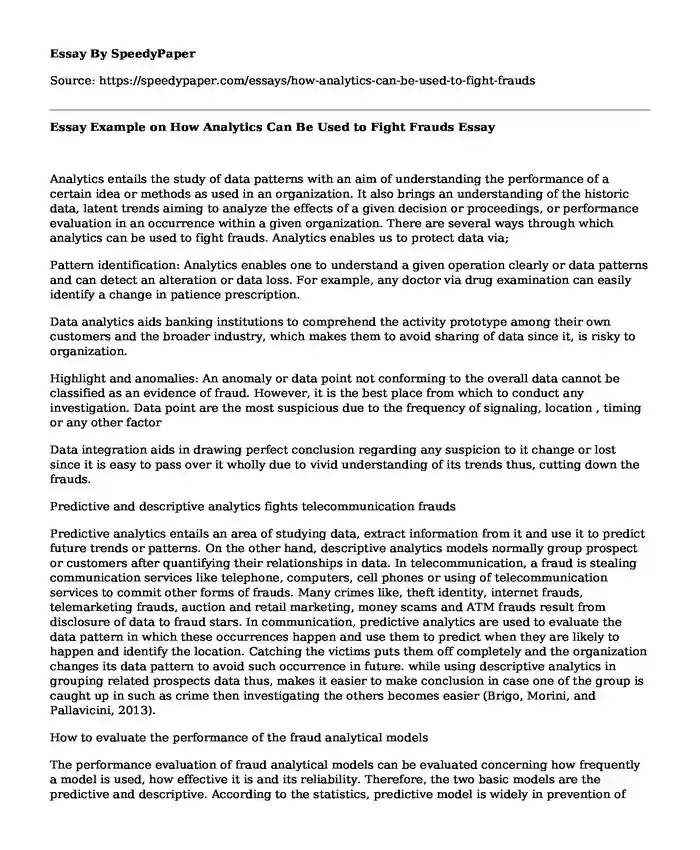
Type of paper:Â | Essay |
Categories:Â | Data analysis Analysis Security |
Pages: | 7 |
Wordcount: | 1805 words |
Analytics entails the study of data patterns with an aim of understanding the performance of a certain idea or methods as used in an organization. It also brings an understanding of the historic data, latent trends aiming to analyze the effects of a given decision or proceedings, or performance evaluation in an occurrence within a given organization. There are several ways through which analytics can be used to fight frauds. Analytics enables us to protect data via;
Pattern identification: Analytics enables one to understand a given operation clearly or data patterns and can detect an alteration or data loss. For example, any doctor via drug examination can easily identify a change in patience prescription.
Data analytics aids banking institutions to comprehend the activity prototype among their own customers and the broader industry, which makes them to avoid sharing of data since it, is risky to organization.
Highlight and anomalies: An anomaly or data point not conforming to the overall data cannot be classified as an evidence of fraud. However, it is the best place from which to conduct any investigation. Data point are the most suspicious due to the frequency of signaling, location , timing or any other factor
Data integration aids in drawing perfect conclusion regarding any suspicion to it change or lost since it is easy to pass over it wholly due to vivid understanding of its trends thus, cutting down the frauds.
Predictive and descriptive analytics fights telecommunication frauds
Predictive analytics entails an area of studying data, extract information from it and use it to predict future trends or patterns. On the other hand, descriptive analytics models normally group prospect or customers after quantifying their relationships in data. In telecommunication, a fraud is stealing communication services like telephone, computers, cell phones or using of telecommunication services to commit other forms of frauds. Many crimes like, theft identity, internet frauds, telemarketing frauds, auction and retail marketing, money scams and ATM frauds result from disclosure of data to fraud stars. In communication, predictive analytics are used to evaluate the data pattern in which these occurrences happen and use them to predict when they are likely to happen and identify the location. Catching the victims puts them off completely and the organization changes its data pattern to avoid such occurrence in future. while using descriptive analytics in grouping related prospects data thus, makes it easier to make conclusion in case one of the group is caught up in such as crime then investigating the others becomes easier (Brigo, Morini, and Pallavicini, 2013).
How to evaluate the performance of the fraud analytical models
The performance evaluation of fraud analytical models can be evaluated concerning how frequently a model is used, how effective it is and its reliability. Therefore, the two basic models are the predictive and descriptive. According to the statistics, predictive model is widely in prevention of fraud from occurring in NGOs, public sectors, banking institutions by analyzing data through recommended software to counter the occurrence of the frauds. Nevertheless, descriptive model is also applicable in handling cases related to frauds in criminal institutions to provide evidence that enables the ruling of the cases. Thus, this model is used by clearly stating the data anomalies and grouping it via different types of softwares used in pattern determination or description.
Key issues in post-processing the fraud analytical models
The key issues in post processing the fraud analytical models are; gaining access to the collect data sources. Some fraud technology system do not account for the quality of data. So, in case these problems are not addressed at first, then the system can be deployed to provide some value. The key step in preparation data for fraud analytics includes; Integration of data of data silos, dealing with missing and erroneous data, entity resolution and processing of unstructured text or information.
Important Challenges
The inability to access data due to privacy concerns is among the few important challenges concerning the fight of frauds. Tight security or high privacy concerns is an important challenge because it does not only prevent the people fighting the frauds but also the fraud stars as well. The other way is failing to discover any kind of relationships in different databases although it could be suspicious. It is important because its complexity exist to anybody who is willing to access it illegally. The last important challenge is use of slow real time search engines. When these engines are, slow they waste time for fraud stars and it is easy to locate the location of their operation. Nevertheless, it is a challenge to fraud investigators since the fraud star can escape while they try to locate their location due to slow internet.
Question 2
Paper Details
Title
What Makes a Solution Implementable in the Real World?
Authors
Nick Cullen
Citation
Nick Cullen. (2014). Approaching 21st Century Education Problems Using Analytics. Informs. Retrieved from https://www.informs.org/About-INFORMS/News-Room/O.R.-Analytics-at-Work-Blog/Approaching-21st-Century-Education-Problems-Using-Analytics [05/03/2015].
The Data Mining Problem Considered
The problem considered was the application of analytic principles in education to increase productivity of the teachers, cut costs of administration to reduce budget and unswerving pressures. There was too much exponential data related to education and had to be collected and sorted in the big data age. Using the required trends to collect information from the available .material with an increasing significance, the process is known as data mining. All the students data collected should not be analyzed any how due to racial differences.
The Data Mining Technique Used
Data mining focused on development of new tools which aided in developing new data patterns. The data patterns were generally the micro concept involved in learning .The research used predictive methodology in building of patterns. There are several researches done by the students and teachers to analyze future trends, for example improvement in education. The writer is addressing the importance of learning analytics in education since its being used to address the future trends affecting education and making improvements where possible.
Predictive Model as Used in the Paper
The students began by learning how to collect data using data mining research knowledge. The predictive methodology is used in arising the trends of data affecting the performance in education. Using the predictive method in analyzing data, the results are positive which means it was effective. For example, higher education levels are adapting use of analytics in improving their services to their customers.
Limitations of this model during the research
The study was limited to some data where the researchers were not supposed to address analytical result regarding racial, religion, gender and social orientation because the general conclusion could be negative to the students. The study also lend to some critics when the performance evaluation of the teachers based on the students standardized students tests result was printed.
Question 3
(a)Sensitivity, specificity and accuracy are described in terms of TP, TN, FN and FP.
Sensitivity = TP/(TP + FN) = (Number of true positive assessment)/(Number of all positive assessment)
Specificity = TN/(TN + FP) = (Number of true negative assessment)/(Number of all negative assessment)
Accuracy = (TN + TP)/(TN+TP+FN+FP) = (Number of correct assessments)/Number of all assessments:
Where: T =True, N=negative, P=positive and F =False;
Accuracy classification
Sensitivity = Tp/(Tp+Fn) = (4440)/(4440-0) = 1
Specificity = Tn/(Tn+Fp) = 1410/(4440+1410) = 0.24
Accuracy = (Tn+Tp)/(Tn+Tp+Fn+Fp) = (1410+4440)/(1410+4440+0+)
5850/(5850+0+0) = 1
(b)The Kolmogorov-Smirnov Curve and its Calculation
It works in a continuous distribution and it is be more sensitive near the centre of the distribution of the series than the tails.
K-S curve limitation is that, the distribution must be fully satisfied and if location, skills, shape parameters are estimated from the data the critical regions of K-S is no longer valid and its typically determined by simulation.
Data CF
50 50
60 110
70 180
80 260
90 350
100 450
110 560
120 680
130 810
140 950
150 1100
Data used:
sensitivity 1-specificity cut-off
0 0 0.1
0.1 0.304 0.3
0.22 0.38 0.3
0.56 0.456 0.5
0.9 0.457 0.9
1 0.458 1
(c)ROC Curve and
ROC curve and estimate the area under the ROC curve
(d)The general similarity about the curve are that both the two curves have formed almost the same curve and due to progressive data.
The two curves formed normal distribution states.
Question 4
Information value of a variable
Information value entails the amount of data that is used to analyze or describe a given variable. Nevertheless, information value can be described as a technique of exploring data to determine which data in a column in a set of data has some predictive power or possess control on a value of a specified autonomous variable. For example, in data mining analysis, assume a credit card company with a pool of customers and wants to investigate about its customers who may not make payment after the investment. For the company to make conclusions on those customers concerning their behavior, it has to observe these traits and analyze their impending actions using these traits. The information in these customers is referred to as the information value, where a customer represents the variable (Weinman, 2012).
Over fitting (decision tree context)
Over fitting refers to problems resulting from errors in data or false relationships. These errors may start from data collection point like keying wrong data for an age trait to a new worker data into the database. Normally, the data or relationship provided is significantly not enough to justify a prediction in an analysis. Spurious relationships may also arise even when the data entered was 100% correct. Wrong data and spurious relationships have the same effect in data mining algorithms. Thus, over fitting can be explained as tremendous occurrence of errors in a decision tree model. A decision tree is used in clear determination of data treads in analytics in data mining practice.
Outlier Truncation
Outliers are values, which looks dubious in the eyes of a researcher. In statistics an outliers is identified as an observation that widely deviates from other observations or normal deviations and may be suspicious, meaning it could have been collected differently from others or there were errors during its placement, (Hawkins, 1980, p.1). For example in a series of statistical data, extreme measurements of values can occur. Incase these data are at the tail or end of the population distribution from which they are drawn, they are known as outliers. While using conventional techniques in measurement identification, if the distance of the mean exceeds the standard deviation of a sample then these measurements are known as the outliers. Thus, outlier truncation is therefore the process of removing an outlier from a given data or sample (Qu and Li, 2013).
EAD in a Basel Context
Exposure at Risk (EAD) is the total value that a bank is exposed to within a given default duration. Every underlying exposure in a bank is given an EAD and it is identified in the internal banks system. However, banks use different default models to calculate the EAD depending with the Internal Rating Board (IRB). With regard to the Basel II context, there are two approaches...
Cite this page
Essay Example on How Analytics Can Be Used to Fight Frauds. (2019, May 14). Retrieved from https://speedypaper.com/essays/how-analytics-can-be-used-to-fight-frauds
Request Removal
If you are the original author of this essay and no longer wish to have it published on the SpeedyPaper website, please click below to request its removal:
- Free Essay on the Book by Brian Greene: The Elegant Universe
- Is Psychology a Science? Free Paper Sample with Annotated Bibliography
- Nursing Essay Sample about Evidence Based Practice Summary
- Essay Sample Behavioral Goals Setting
- Essay Example on the Issues in Sentinel City
- Free Essay Sample: Local News
- Paper Example. The Current Significant Issues Facing Domestic Banks and Their Regulators
Popular categories