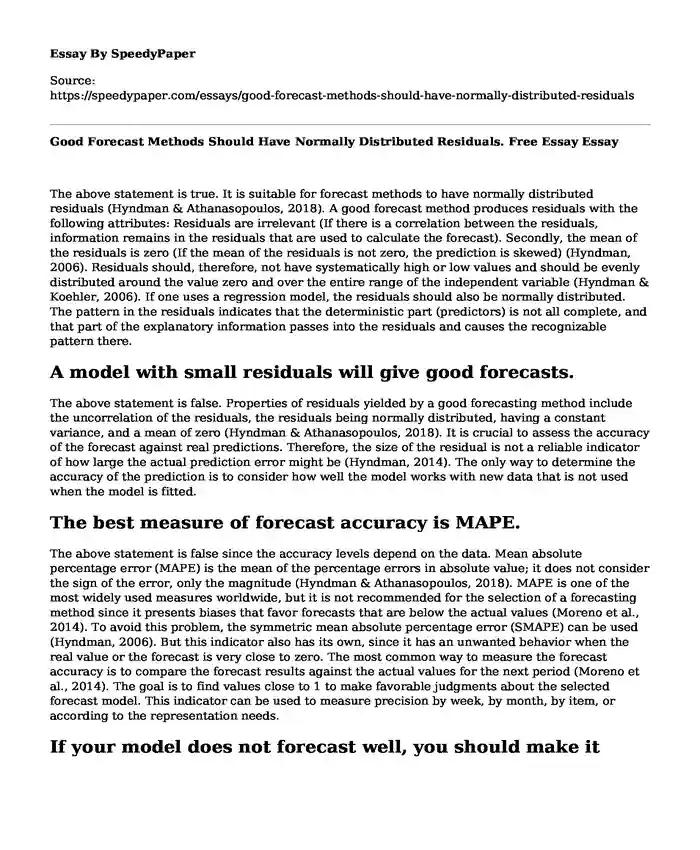
Essay type:Â | Quantitative research papers |
Categories:Â | Economics Mathematics |
Pages: | 4 |
Wordcount: | 1050 words |
The above statement is true. It is suitable for forecast methods to have normally distributed residuals (Hyndman & Athanasopoulos, 2018). A good forecast method produces residuals with the following attributes: Residuals are irrelevant (If there is a correlation between the residuals, information remains in the residuals that are used to calculate the forecast). Secondly, the mean of the residuals is zero (If the mean of the residuals is not zero, the prediction is skewed) (Hyndman, 2006). Residuals should, therefore, not have systematically high or low values and should be evenly distributed around the value zero and over the entire range of the independent variable (Hyndman & Koehler, 2006). If one uses a regression model, the residuals should also be normally distributed. The pattern in the residuals indicates that the deterministic part (predictors) is not all complete, and that part of the explanatory information passes into the residuals and causes the recognizable pattern there.
A model with small residuals will give good forecasts.
The above statement is false. Properties of residuals yielded by a good forecasting method include the uncorrelation of the residuals, the residuals being normally distributed, having a constant variance, and a mean of zero (Hyndman & Athanasopoulos, 2018). It is crucial to assess the accuracy of the forecast against real predictions. Therefore, the size of the residual is not a reliable indicator of how large the actual prediction error might be (Hyndman, 2014). The only way to determine the accuracy of the prediction is to consider how well the model works with new data that is not used when the model is fitted.
The best measure of forecast accuracy is MAPE.
The above statement is false since the accuracy levels depend on the data. Mean absolute percentage error (MAPE) is the mean of the percentage errors in absolute value; it does not consider the sign of the error, only the magnitude (Hyndman & Athanasopoulos, 2018). MAPE is one of the most widely used measures worldwide, but it is not recommended for the selection of a forecasting method since it presents biases that favor forecasts that are below the actual values (Moreno et al., 2014). To avoid this problem, the symmetric mean absolute percentage error (SMAPE) can be used (Hyndman, 2006). But this indicator also has its own, since it has an unwanted behavior when the real value or the forecast is very close to zero. The most common way to measure the forecast accuracy is to compare the forecast results against the actual values for the next period (Moreno et al., 2014). The goal is to find values close to 1 to make favorable judgments about the selected forecast model. This indicator can be used to measure precision by week, by month, by item, or according to the representation needs.
If your model does not forecast well, you should make it more complicated.
The above statement is false. The level of complexity does not determine or guarantee the success of the forecast (Hyndman & Athanasopoulos, 2018). When selecting a forecasting model, one should compare the accuracy against relevance and the cost. It is important to note that the more complicated models are not always more accurate than, the simpler and generally less expensive ones (Hyndman, 2006). One may not have a single criterion to judge the selection of a model in certain situations. In any case, it is necessary to have several of them, and they will always depend on what is going to be forecast and the experience of the forecaster (Hyndman & Koehler, 2006). Depending on the use that is given in the company to short, medium, and long-term predictions, the importance of the forecast horizon must be taken into account when defining a model selection criterion (Hyndman, 2014). A forecasting model that is less effective in the short term and greater in the medium term may be more appropriate and vice versa.
Always choose the model with the best forecast accuracy as measured on the test set.
The above statement is true, but the residuals should be put into consideration as well. The size of the test data set depends on the size of the total sample set and the desired prediction distance, usually 20% of the entire sample set (Hyndman, 2006). Ideally, the test data set should be at least as long as the maximum prediction interval. A prediction model suitable for the training data set may not be able to predict other data sets correctly (Hyndman & Koehler, 2006). Although precision and forecast are essential qualities in the selection of a forecasting model, other factors need to be considered when selecting and evaluating a forecasting model. The characteristics of the information, such as the size or time horizon of the historical data, the behavior of the information, and the type of forecast relationship, should also be considered in the choice.
To assess the accuracy of forecasts, the approaches that can be used to measure the accuracy of forecast models are: How exactly does the model fit the historical data series? How accurately does the model predict the future? As can be deduced from the above, accuracy is a determining factor in the selection process of the forecasting system (Hyndman & Athanasopoulos, 2018). However, a series of information criteria that are very valuable to make predictions with total efficiency and should be considered include the size or time horizon of the data, information behavior, and forecast relationship type (Hyndman, 2006). In the same way, the behavior of the data is also crucial in the search for the forecasting model because if - for example - the historical information presents seasonality, not all the forecasting schemes have this functionality.
References
Hyndman, R. J. (2014). Measuring forecast accuracy. Business forecasting: Practical problems and solutions, 177-183. http://citeseerx.ist.psu.edu/viewdoc/summary?doi=10.1.1.434.2646
Hyndman, R. J. (2006). Another look at forecast-accuracy metrics for intermittent demand. Foresight: The International Journal of Applied Forecasting, 4(4), 43-46. https://robjhyndman.com/papers/foresight.pdf
Hyndman, R. J., & Athanasopoulos, G. (2018). Forecasting: principles and practice. OTexts. https://robjhyndman.com/nyc2018/1-3-ForecastEvaluation.pdf
Hyndman, R. J., & Koehler, A. B. (2006). Another look at measures of forecast accuracy. International journal of forecasting, 22(4), 679-688. https://robjhyndman.com/papers/mase.pdf
Moreno, M., Pol, P., Abad, S., & Blasco, B. (2013). Using the R-MAPE index as a resistant measure of forecast accuracy. Psicothema, 25(4), 500-506. https://pesquisa.bvsalud.org/portal/resource/pt/ibc-115898
Cite this page
Good Forecast Methods Should Have Normally Distributed Residuals. Free Essay. (2023, Nov 27). Retrieved from https://speedypaper.com/essays/good-forecast-methods-should-have-normally-distributed-residuals
Request Removal
If you are the original author of this essay and no longer wish to have it published on the SpeedyPaper website, please click below to request its removal:
- Macroeconomics Research Paper Sample
- Donald Trump Essay Sample from Our Free Database
- Essay Sample on Success and Failures of Dow Chemical Company
- Free Essay about Power Transition: China vs. India
- Essay Sample: Environment Ethical Theories
- Paper Example - Beauveria Bassiana
- Free Essay: Difference between Information and Complexity
Popular categories