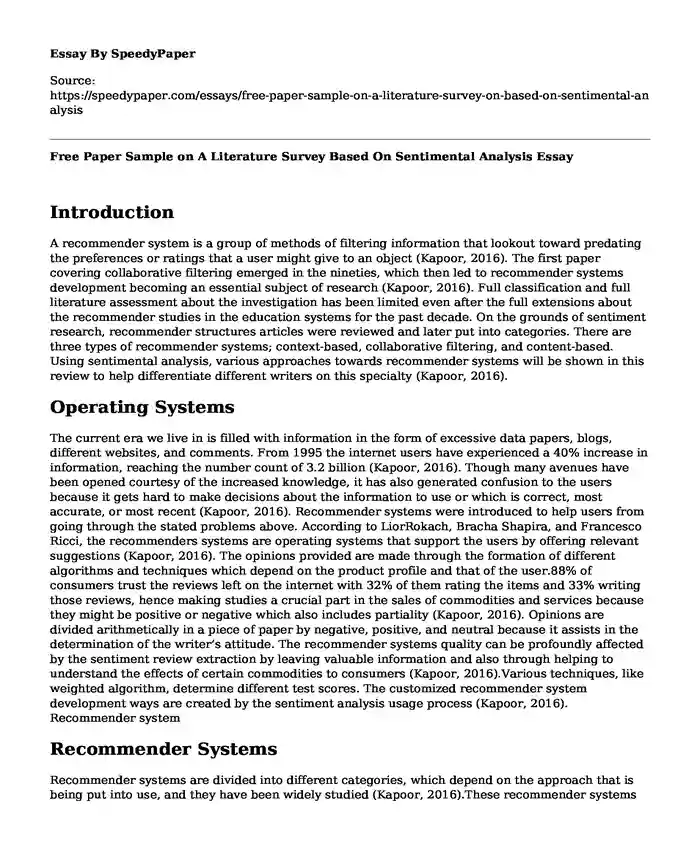
Type of paper:Â | Essay |
Categories:Â | Data analysis Analysis |
Pages: | 6 |
Wordcount: | 1461 words |
Introduction
A recommender system is a group of methods of filtering information that lookout toward predating the preferences or ratings that a user might give to an object (Kapoor, 2016). The first paper covering collaborative filtering emerged in the nineties, which then led to recommender systems development becoming an essential subject of research (Kapoor, 2016). Full classification and full literature assessment about the investigation has been limited even after the full extensions about the recommender studies in the education systems for the past decade. On the grounds of sentiment research, recommender structures articles were reviewed and later put into categories. There are three types of recommender systems; context-based, collaborative filtering, and content-based. Using sentimental analysis, various approaches towards recommender systems will be shown in this review to help differentiate different writers on this specialty (Kapoor, 2016).
Operating Systems
The current era we live in is filled with information in the form of excessive data papers, blogs, different websites, and comments. From 1995 the internet users have experienced a 40% increase in information, reaching the number count of 3.2 billion (Kapoor, 2016). Though many avenues have been opened courtesy of the increased knowledge, it has also generated confusion to the users because it gets hard to make decisions about the information to use or which is correct, most accurate, or most recent (Kapoor, 2016). Recommender systems were introduced to help users from going through the stated problems above. According to LiorRokach, Bracha Shapira, and Francesco Ricci, the recommenders systems are operating systems that support the users by offering relevant suggestions (Kapoor, 2016). The opinions provided are made through the formation of different algorithms and techniques which depend on the product profile and that of the user.88% of consumers trust the reviews left on the internet with 32% of them rating the items and 33% writing those reviews, hence making studies a crucial part in the sales of commodities and services because they might be positive or negative which also includes partiality (Kapoor, 2016). Opinions are divided arithmetically in a piece of paper by negative, positive, and neutral because it assists in the determination of the writer’s attitude. The recommender systems quality can be profoundly affected by the sentiment review extraction by leaving valuable information and also through helping to understand the effects of certain commodities to consumers (Kapoor, 2016).Various techniques, like weighted algorithm, determine different test scores. The customized recommender system development ways are created by the sentiment analysis usage process (Kapoor, 2016). Recommender system
Recommender Systems
Recommender systems are divided into different categories, which depend on the approach that is being put into use, and they have been widely studied (Kapoor, 2016).These recommender systems are categorized thrice like stated earlier into content, context-based, and collaborative filtering, which will be analyzed throughout the paper (Kapoor, 2016).
Collaborative Filtering
This system I based on the user's historical data that is already available by using the same user's numerical reviews that were made by them (Kapoor, 2016). The user profile is created by historical data found while the item profile is created by the item historical data (Kapoor, 2016). In this case, the system recommendation is made by both patterns. The Netflix competition has significantly populated collaborative filtering. In finding suggestions and predations towards sales and products, collaborative filtering has been considered to be the easiest and most common. However, it has some limitations, which has led to new techniques and methods being developed (Kapoor, 2016).
This system helps people through the use of unknown preferences. Still, with the help of other user preferences, the similarity between the user and product profiles is computed by the method of Pearson and cosine similarity approach (Kapoor, 2016).The main limitation of this system is the limitation of data information expandability and the problem to a cold start. Three algorithms are introduced in this system, which is the main. They are memory-based, hybrid CF, and model-based, and they join the other recommendation systems with collaborative filtering and their capability to deal with challenges (Kapoor, 2016).
Memory-Based Collaborative Filtering
Memory-based collaborative filtering is represented by item-based and user-based collaborative filtering, making it easy for implementation, and the correlated items also scale properly (Kapoor, 2016). In this system, the content of the things getting considered does not matter and does not get any consideration. There is limited data in this system, which also has a cold start and human rating dependency, which makes it weak or unreliable (Kapoor, 2016).
Model-Based Collaborative Filtering
In this model, I recognize intricate patterns that depend entirely on training data through the designing and development of models like machine learning and data mining algorithms (Kapoor, 2016). Data available for everyone to see is then provided for tasks that concern collaborative filtering by cognitive filtering of the data that come from the learned models (Kapoor, 2016). This system offers integrated reasoning for the recommendation, and the process of model building is relatively expensive. The system is also fond of letting go of crucial data for measurement cutting techniques, which is its most significant liability (Kapoor, 2016).
Hybrid Collaborative Filtering Techniques
In this system, there is a combination of different recommender systems and collaborative approaches in various techniques to acquire better outcomes than another collaborative filtering (Kapoor, 2016).In this approach, limitations found in model-based and memory-based collaborative filtering like the limitation of data, cold start, and expandability. The various recommender techniques that can be combined with CF to achieve the best outcomes are mostly the content-based approaches (Kapoor, 2016). Users are part of a group after those that have the same interests are put together.
Content-Based Recommender Filtering
It is a system that is based solely on content, and therefore its focus is no product features and creates the profile of the user by use of previous reviews. While creating that of the product depends on the features and the reports that it has gained earlier (Kapoor, 2016). The users' opinion is issued in pairs and features of the product on the reviews where the reviews of the user have the product feature and their sentiment towards it (Kapoor, 2016). This system helps overcome the problem of sparseness found in collaborative filtering (Kapoor, 2016).
Content-Based Collaborative Filtering
In this technique, the profiles of the users themselves are taken into context or consideration. The methods help show the actual interest a user has and assist in predicting what they might want to do at any given time; it accurately indicates the users' interest (Kapoor, 2016). This system is condition to adapt to the likes and dislikes of the users through its adaptive filtering. It provides a clear comparison between the content provided by the users’ interests and the materials contained in the item description (Kapoor, 2016). This system is preferable because better profiles for new users are done by collecting information gathered from old users (Kapoor, 2016). The primary purpose of this system or what makes it better than the earlier stated above is the ability to compare content base and pure collaborative filtering. However, it has a limitation in the scarcity of data provided (Kapoor, 2016).
Context-Based Collaborative Filtering
Expansion of the items' convection to certain conditions to assimilate the user dependency on information is achievable by this recommender system (Kapoor, 2016). This is essential in leaving the cumbersome process that forces users to fill in personal details in huge numbers (Kapoor, 2016).
Context-Based Recommendation System
Improvement of the recommender systems using collaborative filtering technique which dwell on items features and contents is possible. It can be done because it has earlier attained notable success in the past (Kapoor, 2016). Personalization of a higher level is a requirement to provide more accurate suggestions to the recommender systems, which entails recognizing user information when designing the system (Kapoor, 2016). This system is essential in helping the system be more active in providing more information about users or different people with different preferences. Various techniques are used by a context-based recommendation system to acquire situational information about the users without disturbing them (Kapoor, 2016). The users' data can be obtained from the devices they are using, which shows even the time of the day, which is very helpful in the provision of recommendations. The information derived from the user’s device location can provide so much information essential even for tourists, like providing the weather condition and route (Kapoor, 2016).
Conclusion
Parts of records in excess occurrences on the internet are addressed by the recommender structures (Kapoor, 2016). The systems have evolved at the same rate in which the internet is growing hence recent updates to support efficiency and accuracy of recommendations. Sentimental analysis is still ongoing to develop even more accurate recommender systems (Kapoor, 2016). The recommender system has been proven to be a crucial part of item purchases (Kapoor, 2016).
References
Jain, A., Jain, V., & Kapoor, N. (2016). A literature survey on the recommendation system based on the sentimental analysis. An International Journal (ASCII), 3(1). https://www.academia.edu/download/42598847/3116acii03.pdf
Cite this page
Free Paper Sample on A Literature Survey Based On Sentimental Analysis. (2023, Nov 06). Retrieved from https://speedypaper.com/essays/free-paper-sample-on-a-literature-survey-on-based-on-sentimental-analysis
Request Removal
If you are the original author of this essay and no longer wish to have it published on the SpeedyPaper website, please click below to request its removal:
- Essay Example: Cash Flow and Profit Margin Study
- European Perception in the East Africa and the World
- Resistance to Civil Government by Henry David Thoreau - Essay Example
- Paper Example: Evolutionary Biology
- Essay Sample on Benefits of Microcirculation
- Paper Example. Effects of Acid Rain on the Environment
- Paper Example. Anatomy and Physiology
Popular categories