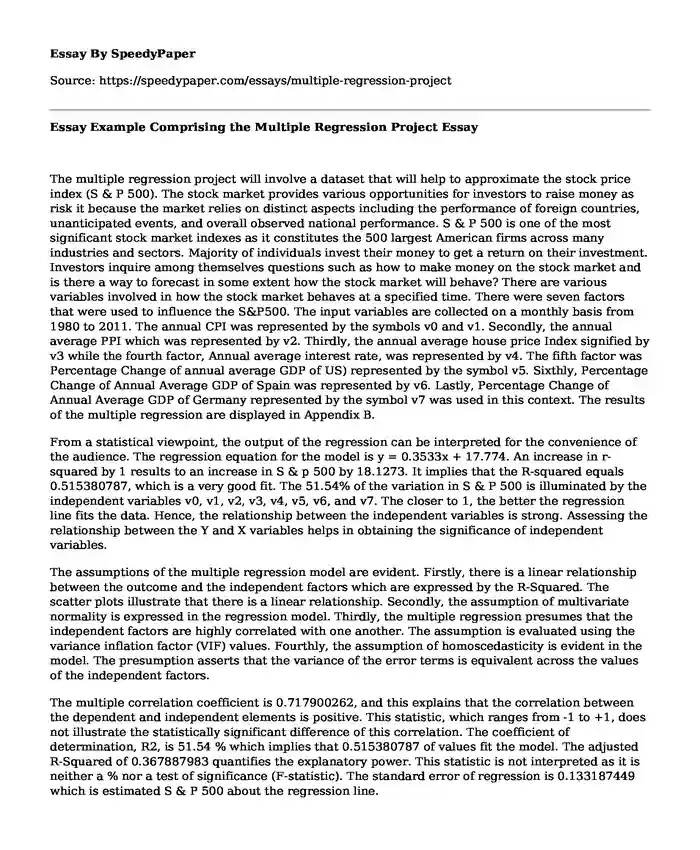
Type of paper:Â | Essay |
Categories:Â | Data analysis Statistics |
Pages: | 5 |
Wordcount: | 1374 words |
The multiple regression project will involve a dataset that will help to approximate the stock price index (S & P 500). The stock market provides various opportunities for investors to raise money as risk it because the market relies on distinct aspects including the performance of foreign countries, unanticipated events, and overall observed national performance. S & P 500 is one of the most significant stock market indexes as it constitutes the 500 largest American firms across many industries and sectors. Majority of individuals invest their money to get a return on their investment. Investors inquire among themselves questions such as how to make money on the stock market and is there a way to forecast in some extent how the stock market will behave? There are various variables involved in how the stock market behaves at a specified time. There were seven factors that were used to influence the S&P500. The input variables are collected on a monthly basis from 1980 to 2011. The annual CPI was represented by the symbols v0 and v1. Secondly, the annual average PPI which was represented by v2. Thirdly, the annual average house price Index signified by v3 while the fourth factor, Annual average interest rate, was represented by v4. The fifth factor was Percentage Change of annual average GDP of US) represented by the symbol v5. Sixthly, Percentage Change of Annual Average GDP of Spain was represented by v6. Lastly, Percentage Change of Annual Average GDP of Germany represented by the symbol v7 was used in this context. The results of the multiple regression are displayed in Appendix B.
From a statistical viewpoint, the output of the regression can be interpreted for the convenience of the audience. The regression equation for the model is y = 0.3533x + 17.774. An increase in r-squared by 1 results to an increase in S & p 500 by 18.1273. It implies that the R-squared equals 0.515380787, which is a very good fit. The 51.54% of the variation in S & P 500 is illuminated by the independent variables v0, v1, v2, v3, v4, v5, v6, and v7. The closer to 1, the better the regression line fits the data. Hence, the relationship between the independent variables is strong. Assessing the relationship between the Y and X variables helps in obtaining the significance of independent variables.
The assumptions of the multiple regression model are evident. Firstly, there is a linear relationship between the outcome and the independent factors which are expressed by the R-Squared. The scatter plots illustrate that there is a linear relationship. Secondly, the assumption of multivariate normality is expressed in the regression model. Thirdly, the multiple regression presumes that the independent factors are highly correlated with one another. The assumption is evaluated using the variance inflation factor (VIF) values. Fourthly, the assumption of homoscedasticity is evident in the model. The presumption asserts that the variance of the error terms is equivalent across the values of the independent factors.
The multiple correlation coefficient is 0.717900262, and this explains that the correlation between the dependent and independent elements is positive. This statistic, which ranges from -1 to +1, does not illustrate the statistically significant difference of this correlation. The coefficient of determination, R2, is 51.54 % which implies that 0.515380787 of values fit the model. The adjusted R-Squared of 0.367887983 quantifies the explanatory power. This statistic is not interpreted as it is neither a % nor a test of significance (F-statistic). The standard error of regression is 0.133187449 which is estimated S & P 500 about the regression line.
The variance analysis section offers an evaluation of the cumulative variation of the dependent variable, in this case, study (the projected value of S & P 500 ) into the addressed and unaddressed portions. The SS regression (0.43389239) elucidates the variation addressed by the regression line. SS residual (0.407994621) is the incongruity of the dependent variable that is not explained.
The F-Statistic is quantified using the percentage of the MS regression to the MS residual. This statistic is then compared with the critical F-Value to assessment the null hypothesis. The p-value linked with the calculated surpass the calculated F-Value. Offering a comparison of this value with 5%, for instance, evaluates rejection of the null hypothesis.
The results of the estimated regression constituted the predicted parameters of the t-statistic, the standard error of the parameter, the corresponding p-value and the bounds of 95% and 90 % confidence intervals. The explanatory element is the seven factors that are t-statistic and less than a 5% significance level that surpass the critical values. The relationship between independent variables and S & P 500 won is positive. The coefficient is 0.331186474 which implies that an increase in the dependent variables increases the S & P 500 by 0.331186474. CPI, US PPI, HPI, Maturity Rate, US GDP, Spain GDP, Germany GDP are positively correlated with the projected S & P 500 essential in stock market returns (Bolin et al., 2013).
Introduction of an interaction term to a regression model can help simplify the understanding of the relationships among the factors and enable more testing of the hypothesis. A variable can be eliminated to improve the model.
The stepwise selection regression of the dataset does not improve the model. The R2 are biased high 0.363, 0.509, and 0.561. The F-Statistics of 21.690, 19.195, and 15.343 do not have a claimed distribution. The standard errors of the coefficients are too small (0.138426230, 0.123171454, and 0.118081723). The confidence intervals around the coefficients are too narrow (0.1 and 0.05). The p-values are low because of various comparisons and are problematic to rectify. The issue of collinearity is exacerbated.
Forward selection is a form of stepwise regression which starts with an empty model and includes variables in order. In every forward step, the statistician is bound to include one variable that offers the single best enhancement in the model. This regression does not improve the model. The regression has lower adjusted and predicted R-squared values. Adjusted R-squared lowers when a new variable does not improve the model by more than likelihood. The predicted R-Squared is a cross-validation technique that can also reduce. The cross-validation partitions help to evaluate whether the model is generalizable in the dataset. The adjusted R-Squared value is 0.347, 0.483 and 0.525.
The backward selection does not improve the model. This form of regression guarantees the similar optimality of the large subsets though might yield very poorly performing small subsets. The regression has lower adjusted and predicted R-squared values. These values become lower when a new variable does not enhance the model by more than chance. The values of the adjusted R-Squared value are 0.516, 0.531, 0.542, and 0.542
The chosen model is the second regression model that involves the inclusion of the dummy variable and interaction term. The intercept is the expected mean value of Y when all X equals to zero. It is important to start with a regression equation with a solitary predictor. If x sometimes is equivalent to zero, then the intercept is typically the anticipated mean value of Y at that value. If x is never equivalent to zero, then the slope has no intrinsic connotation. The motive of a regression model is to grasp the association between the predictors and the response. In case so, and in case x is never equivalent to zero, there is no interest in the intercept. It does not address anything regarding the relationship between X and Y.
I am comfortable with the model because of the large R-Squared implying low multi-collinearity. Changing the sample size of data set from 40 to 55 observations would be the top priority if permitted to do so. The statistician can rebuild the regression model by getting rid of highly correlated predictors from it. Secondly, in case there are at least two elements with a high VIF, it is important to remove it from the model. Finally, the statistician can utilize the Partial Least Squares Regression (PLS) or Principal Components Analysis to enhance the model. These regression methods reduce the number of predictors to a smaller sequence of the uncorrelated component.
Work Cited
Bolin, Jocelyn H. "Hayes, Andrew F.(2013). Introduction to Mediation, Moderation, and Conditional Process Analysis: A RegressionBased Approach. New York, NY: The Guilford Press." Journal of Educational Measurement 51.3 (2014): 335-337.
APPENDIX
Appendix A
Year S&P500 US US US US US SPAIN GERMANY
Change CPI PPI HPI Maturity Rate GDP GDP GDP
1978 0.153153 109.6 56.0986 135.6 0.0885 0.0451 0.0093 0.0511
1979 0.03125 113.6 57.48551 144.33 0.0849 0.0425 -0.0103 0.0192
1980 0.112225 82.4 48.15998 102.71 0.1143 0.0962 0.0221 0.0101
1981 0.199279 90.9 52.62937 107.79 0.1392 0.0969 -0.0013 0.0053
1982 -0.11805 96.5 54.72269 111.5 0.1301 0.0379 0.0125 -0.0039
1983 0.230179 99.6 55.62113 115.8 0.111 0.1139 0.0177 0.0157
1984 0.153153 103.9 56.78408 120.81 0.1246 0.0926 0.0178 0.0282
1985 0.03125 107.6 57.27663 126.97 0.1062 0.0737 0.0232 0.0233
1986 0.213287 109.6 56.0986 135.6 0.0767 0.0486 0.0325 0.0229
1987 0.270413 113.6 57.48551 144.33 0.0839 0.0757 0.0555 0.014
1988 -0.05293 118.3 59.49463 152.04 0.0885 0.0776 0.0509 0.0371
1989 0.139321 124 62.48219 160.05 0.0849 0.0648 0.0483 0.039
1990 0.191205 130.7 65.23701 165.04 0.0855 0.0451 0.0378 0.0526
1991 -0.04259 136.2 66.05396 168.17 0.0786 0.0425 0.0255 0.0511
1992 0.278319 140.3 66.89465 172.87 0.0701 0.0666 0.0093 0.0192
1993 0.046025 144.5 67.89209 176.88 0.0587 0.05 -0.0103 -0.0096
1994 0.086759 148.2 68.79928 181.41 0.0709 0.0631 0.0238 0.0246
1995 -0.01636 152.4 70.80365 186.94 0.0657 0.0432 0.0276 0.0174
1996 0.320623 156.9 72.43279 193.43 0.0644 0.0625 0.0267 0.0082
1997 0.247062 160.5 72.67027 199.95 0.0635 0.0605 0.0369 0.0185
1998 0.257289 163 71.90083 210.2 0.0526 0.0611 0.0431 0.0198
1999 0.296265 166.6 73.112 220.55 0.0565 0.0644 0.0448 0.0199
2000 0.141595 172.2 76.09006 234.66 0.0603 0.055 0.0529 0.0296
2001 -0.0631 177.1 76.68851 252.2 0.0502 0.0219 0.04 0.017
2002 -0.14631 179.9 76.1803 268.16 0.0461 0.0376 0.0288 0
2003 -0.21432 184 78.10867 284.85 0.0401 0.0642 0.0319 -0.0071
2004 0.264199 188.9 81.46671 311.47 0.0427 0.0631 0.0317 0.0117
2005 0.043169 195.3 85.95992 346.78 0.0429 0.0652 0.0372 0.0071
2006 0.082376 201.6 89.43668 371.6 0.048 0.0512 0.0417 0.037
2007 0.11373 207.3 92.85172 375.69 0.0463 0.044 0.0377 0.0326
2009 -0.3722 214.537 95.25031 336.68 0.0326 0.0011 -0.0357 -0.0562
2010 0.298066 218.056 100 322.88 0.0322 0.0456 0.0001 0.0408
2011 0.141548 224.939 107.78 310.5 0.0278 0.0364 -0.01 0.0366
2012 0.264199 188.9 81.46671 311.47 0.0427 0.0631 0.0317 0.0117
2013 0.043169 195.3 85.95992 346.78 0.0429 0.0652 0.0372 0.0071
2014 0.082376 201.6 89.43668 371.6 0.048 0.0512 0.0417 0.037
2015 0.11373 207.3 92.85172 375.69 0.0463 0.044 0.0377 0.0326
2016 -0.3722 214.537 95.25031 336.68 0.0326 0.0011 -0.0357 -0.0562
2017 0.298066 218.056 100 322.88 0.0322 0.0456 0.0001 0.0408
2018 0.141548 224.939 107.78 310.5 0.0278 0.0364 -0.01 0.0366
Cite this page
Essay Example Comprising the Multiple Regression Project. (2022, Oct 18). Retrieved from https://speedypaper.com/essays/multiple-regression-project
Request Removal
If you are the original author of this essay and no longer wish to have it published on the SpeedyPaper website, please click below to request its removal:
- Essay Example: Personal Selling in Death of a Salesman
- Ignite-Style Expertise Speech Example on the French Horn
- Free Essay Comprising the Full Script Analysis of Cloud 9 by Carl Churchill
- A Visit to Newgate, Essay Example for Everyone
- Free Essay Describing the Case Study of Electrolyte Imbalances
- Free Essay - Terminating Employer-Employee Relationship
- Essay Sample on Sexual Assault on Campus
Popular categories